Statistical Tools and their Usage - Factor Analysis
Factor Analysis is a data reduction technique. Given a large number of attributes, factor analysis identifies a few underlying dimensions by grouping the attributes based on the correlation between the attributes. For example, price of a product, the cost after sales service, and maintenance expense can be identified as a part of single dimension: Total Cost
Diagrammatic Representation
Attributes such as Insomnia, Nausea and Suicidal Tendency can be collectively described by a single term: Depression. Here three variables/attributes have been represented by a single factor.
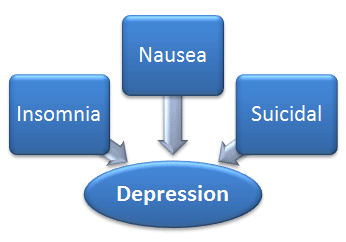
Implementations of Factor Analysis
Factor analysis is usually more meaningful when it is followed by subsequent procedures such as clustering. Following are some of the applications of this method:
- Recognition of underlying factors: Bundles homogenous variables into new sets (i.e. factors)
- Screening variables: Factor analysis associates one variable as a representation of several other similar variables which is further useful for regression analysis.
- Cluster analysis: Helps couple objects into categories contingent on their factor scores.
Factoring in Marketing Research
While factor analysis is used in several fields such as psychometrics, psychology, physical sciences, etc, we will focus on the topic relevant in this module: marketing research. The procedure for utilizing factor analysis in a typical marketing research study is as follows:
- Enlist all the attributes consumers might consider while buying a product or category.
- Collect responses from consumers through quantitative data collection techniques such as surveys on the basis of these attributes.
- Feed in the collected data in a statistical program to come up with fewer factors that are a representation of all the important attributes enlisted above. SPSS is the most commonly deployed software for factor analysis.
- Further utilize these factors for building perceptual maps, product positioning, regression, clustering, etc as per the purpose of the study.
Industry examples requiring usage of this tool
- Consumers rate a product on 20 different attributes. What are basic dimensions underlying these 20 attributes?
- Customers rate the importance of different attributes for a service. Can we identify the underlying dimensions of service?
Factor Analysis Assumptions
For this method to be valid, certain assumptions must hold true:
- No outliers in the data set: This means that no extreme data should be present in the data set. For example, consider the following values: 1, 5, 8,-4, 23, and 1246783. The latter is an outlier that overwhelms the remaining data.
- Adequate sample size: This simply implies that there must be more variables that factors. This is intuitive because 3 variables cannot produce 4 factors. Also, each variable must have more data values than the number of factors.
- The data sets must possess no perfect multicollinearity
Var1 Var2 Var3 1 2 3 2 4 6 3 6 9 4 8 12
Each variable must be unique. Var2 and Var3 can be reduced to Var1 by dividing them by numbers 2 and 3 respectively. Since they are basically the same data sets, they cannot be used for forming factors.
❮❮ Previous | Next ❯❯ |
Related Articles
- Conjoint Analysis - Meaning, Usage and its Limitations
- Sources of Data
- What is Big Data ?
- Big Data and the Power to Predict
- Attitude Measurement Scales
Authorship/Referencing - About the Author(s)
![]()
The article is Written and Reviewed by Management Study Guide Content Team. MSG Content Team comprises experienced Faculty Member, Professionals and Subject Matter Experts. We are a ISO 2001:2015 Certified Education Provider. To Know more, click on About Us. The use of this material is free for learning and education purpose. Please reference authorship of content used, including link(s) to ManagementStudyGuide.com and the content page url.